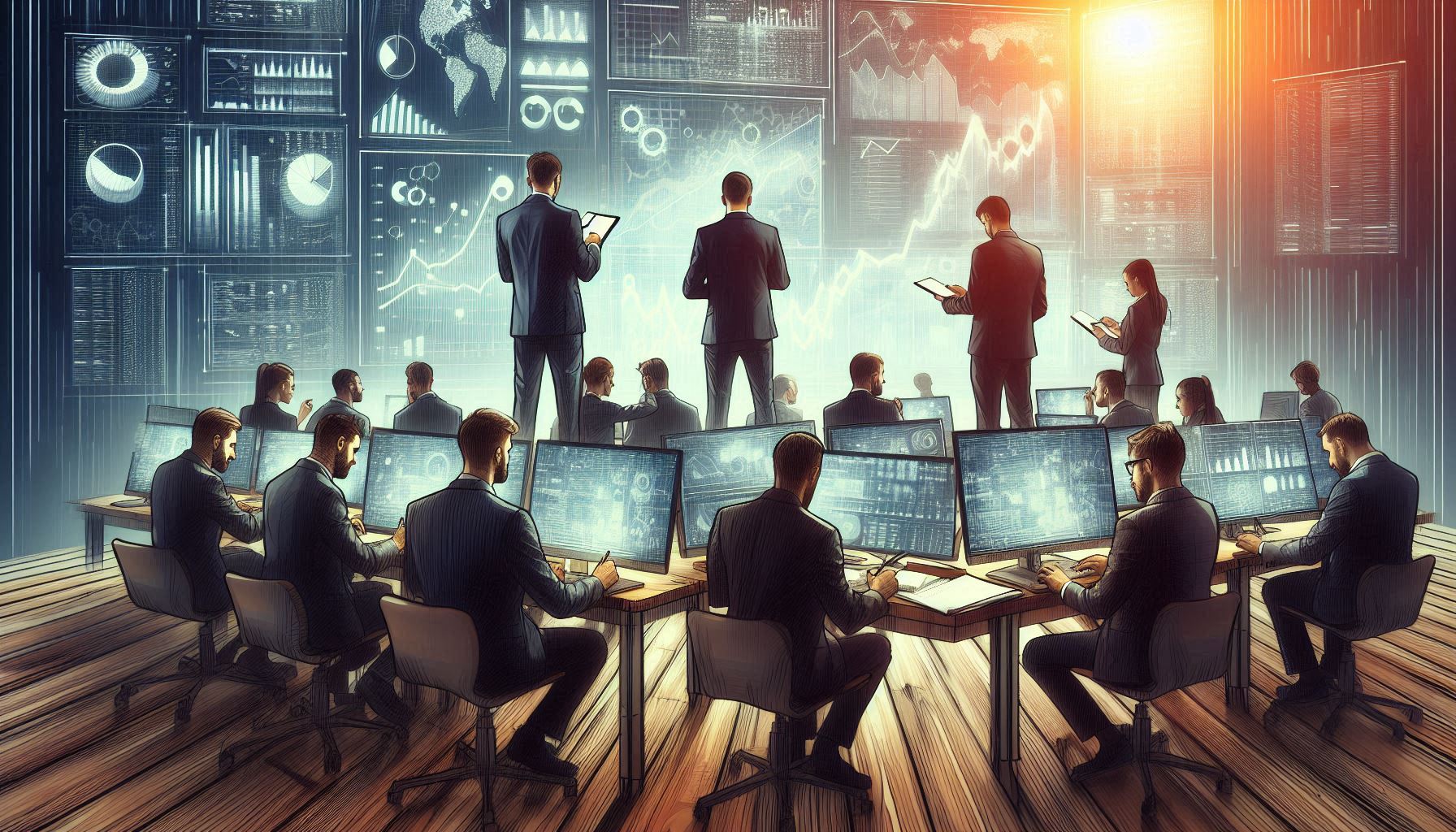
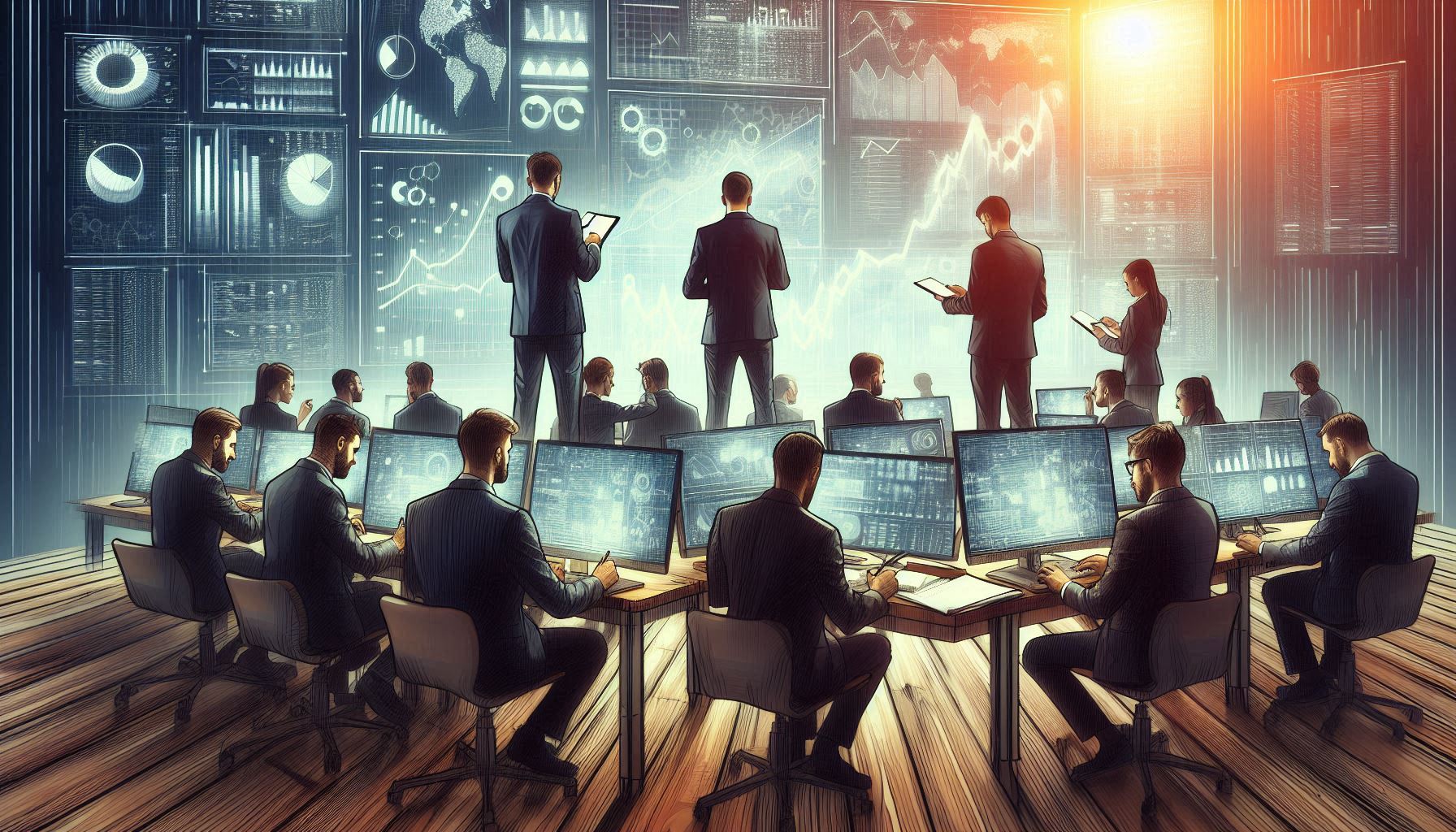
Good data quality is crucial for successful data and analytics initiatives and is increasingly pivotal to artificial intelligence impact.
D&A leaders, including chief data and analytics officers, are also having to contend with an ever-more complex business environment, with ever-more complex data needs and challenges. This is being further compounded by the growing need for AI-ready data.
The demand for AI-ready data is adding fresh impetus to investment in data quality improvement, although making the business case for this is notoriously challenging. With this in mind, D&A leaders must take tangible and pragmatic steps to understand, address and control data quality on an ongoing basis.
Below are five key steps D&A leaders can take to build a business case for continuous data quality assurance, in order to deliver on the promise of business value through their data, analytics and AI investments.
In order to motivate business leaders to improve data quality, D&A leaders must demonstrate its impact on achieving specific business outcomes.
Data-driven evidence is needed to prove the impact of poor data quality on business outcomes. Starting with the critical business processes and KPIs needed to deliver strategic business outcomes, D&A leaders should identify the data that is needed and where it comes from.
Next, they can use data quality profiling to analyze the quality of critical data elements in key applications, and demonstrate their impact on KIs and business performance. Explore the results of data quality profiling with business stakeholders by investigating how data quality limitations impede business performance, and how improvements could contribute to better top-line outcomes.
Finally, avoid attributing data quality issues to a single cause. Instead, explore root causes across people, processes, technology, organization, governance and culture. This analysis will inform a targeted approach to systemic data quality improvement.
There are three main ways that D&A leaders can identify the root causes of poor data quality and develop a practical scope for data quality improvement.
The first is by analyzing the data journey of critical data elements contributing to business outcomes. Understanding the story of these data elements as they flow through the enterprise will uncover the contributing factors to poor data quality.
The second is by identifying the impact, opportunity and risk of each contributing factor of poor data quality. For each of these aspects, evaluate factors contributing to poor data quality by assessing their impact, potential opportunities to scale business value and the risk they pose.
Finally, D&A leaders can triage the solution scope based on critical data quality factors. They can analyze the practicality of addressing all of the factors they have identified that impact data quality.
To build a solution proposal as a collaborative process, D&A leaders can:
Figure 1
A good business case must identify the anticipated benefits of the initiative, and it must be tangible, quantifiable and desirable to the stakeholders, with the causal contributions identified.
D&A leaders must position this data quality investment as the foundation for future data quality improvements needed for other business outcomes. Prior to presenting the final business case to senior business stakeholders, the logic behind the business case should be reviewed.
To do this, D&A leaders can assess their data quality improvement proposals total cost of ownership , focusing on detailed financial estimates for the recommended option if stakeholders have been involved throughout.
They can also develop a ROI model by taking into account the best-case, most likely and worst-case scenarios that could have an impact on costs, and provide upper, middle and lower thresholds for cost estimates.
Finally, D&A leaders can create a story that demonstrates how this data quality investment can be leveraged to address other business challenges. They can develop a story arc for business cases that not only addresses the question “What is the business value of data quality improvement in scope?” but also includes and finishes on the question “What comes next?”
By following these steps, D&A leaders can build a compelling business case for continuous data quality assurance, ensuring ongoing improvement and alignment with organizational goals.
Saul Judah is a VP analyst within the Analytics Apps and Governance team at Gartner Inc., focusing on information governance, data quality and information strategy. Judah works with Gartner clients on establishing and running effective information governance programs and creating and delivering data quality strategies. He wrote this article for SiliconANGLE. Gartner analysts will provide additional analysis on data, analytics and AI at Gartner Data & Analytics Summit March 3-5 in Orlando, Florida.
THANK YOU