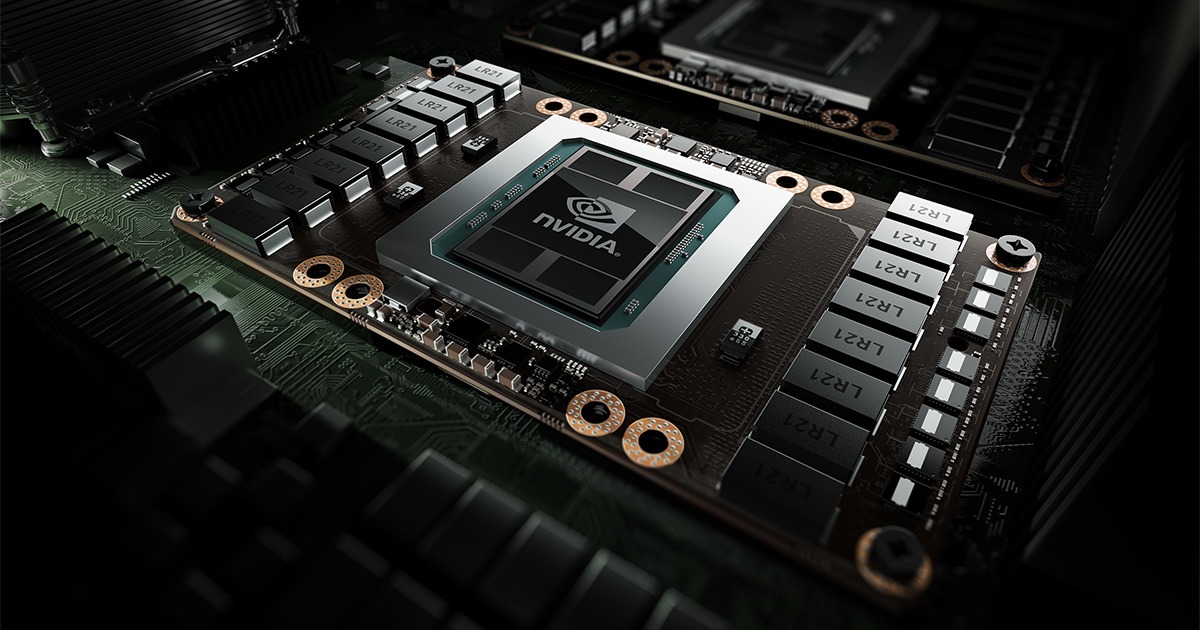
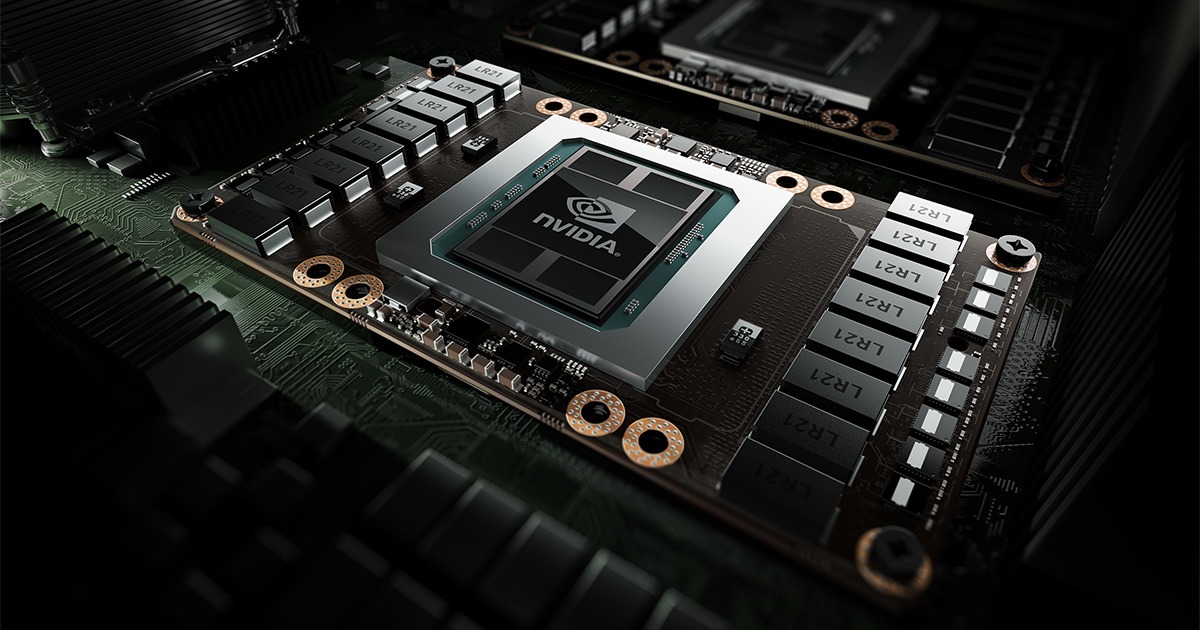
Technology stocks were hammered in early Monday trading on news that a Chinese startup has built and released to open source a chatbot based on an artificial intelligence model that rivals the performance of the most capable models built by U.S. companies but at a fraction of the cost.
DeepSeek, which is operated by Hangzhou DeepSeek Artificial Intelligence Co. Ltd. and Beijing DeepSeek Artificial Intelligence Co. Ltd., made waves last week when it published a paper outlining the development process for its two primary models called DeepSeek-R1-Zero and DeepSeek-R1.
The R1 model is reported to have been trained for just $5.6 million, a stark contrast to the hundreds of millions or billions spent by U.S. companies such as OpenAI LLC, Google LLC, and Meta Platforms Inc. The cost advantage suggests that significant progress can be made without the massive capital expenditures that have been a cornerstone of AI development.
DeepSeek’s claims that it needs fewer and less advanced chips than other AI models immediately raised doubts about whether the massive spending on artificial intelligence that is expected over the next few years is necessary.
Stocks of U.S. companies with heavy investments in AI were hit hard on Monday, led by Nvidia Corp., which was down more than 15% at noon. Shares of other AI-focused chipmakers were also slammed. Broadcom Inc. fell 16%, Taiwan Semiconductor Manufacturing Corp. dropped more than 14% and Marvell Technology Inc. sank 14%. The Nasdaq Composite Index was off more than 3% in early trading.
Meanwhile, DeepSeek quickly rocketed up the charts to become the No. 1 productivity app on the Apple App Store.
The market reaction reflects anxiety over whether the U.S. can maintain its dominance in AI technology amid growing competition. The advancements sow doubts about the “need for huge western hardware investment,” wrote the Financial Times.
Writing on X, venture capitalist Marc Andreessen called Deepseek R1 “AI’s Sputnik moment,” referring to the Soviet Union’s surprise 1957 launch of a satellite that kicked off the space race. He added that the model is “one of the most amazing and impressive breakthroughs I’ve ever seen.”
However, Gartner Inc. VP Analyst Chirag Dekate characterized the stock market selloff as a “momentous overreaction.” Dekate, who holds a Ph.D. in computer science, read the paper published by DeepSeek researchers and said it reveals important innovations in areas such as memory management and the performance tuning strategy called key-value cache optimization but indicates no fundamental breakthroughs.
“It is not the case that DeepSeek has somehow developed an innovative technique that eliminates the need to use acceleration for training or inferencing models,” he said. Investors are misinterpreting DeepSeek’s claims of a breakthrough in processing efficiency as indicating that graphic processing units and other accelerators will be less important in the future. “You still need underlying GPU infrastructures to scale models,” he said. “Tomorrow’s innovations will require more of them, not less. This does not change anything from that perspective.”
China is regarded as the U.S.’s biggest competitor in AI, but China’s competitive position has been hobbled by difficulty obtaining high-end chips, many of which are covered by export controls. DeepSeek’s ability to achieve comparable performance to the largest and costliest AI models may now force investors and chipmakers to rethink their approach to AI investment.
But some analysts note that U.S. companies still hold a strong position in AI and have invested heavily in hardware infrastructure. “Markets had gotten too complacent on the beginning of the Trump 2.0 era and may have been looking for an excuse to pull back,” Michael Block, a market strategist at Third Seven Capital LLC, told CNN.
Others see DeepSeek’s cost advantages accelerating AI adoption. “This cost advantage opens the door to unmetered and pervasive access to AI, which is sure to be both exciting and highly disruptive,” said Ted Miracco, CEO of mobile app security vendor Approov Ltd.
Azeem Azhar, creator of the influential Exponential View Substack, wrote in his newsletter that DeepSeek’s innovations in reducing training and inferencing costs will accelerate AI adoption, open new classes of applications based on adversarial training techniques and spur further innovations in open-source.
It could also attract talented computer scientists to occupations other than finance. “We are seeing the smart kids working in finance move into the real economy,” he wrote. “Look what happens when your best and brightest do something other than chasing arbitrage.”
Nvidia issued a low-key statement of support, complimenting DeepSeek on “an excellent AI advancement and a perfect example of Test Time Scaling,” which is a technique used to improve performance in memory-constrained conditions. “DeepSeek’s work illustrates how new models can be created using that technique, leveraging widely available models and compute that is fully export control compliant,” a spokesman said.
The news comes as U.S. companies and the U.S. government have signaled their intentions to step up spending on AI. Last week a consortium of companies announced plans to invest $500 billion to build a cluster of AI data centers. Meta Platforms last week also said it plans to spend $65 billion this year to expand AI infrastructure.
“Companies relying on brute force, pouring unlimited processing power into their solutions, remain vulnerable to scrappier startups and overseas developers who innovate out of necessity,” said Mali Gorantla, chief scientist at AI governance firm AppSOC Inc.
Keith Lerner, an analyst at Truist Financial Corp., told CNN, “The DeepSeek model rollout is leading investors to question the lead that U.S. companies have and how much is being spent and whether that spending will lead to profits (or overspending).”
In their paper posted on arXiv, a consortium of authors explained how for two primary models — DeepSeek-R1-Zero and DeepSeek-R1 – were initially trained using via large-scale reinforcement learning without the initial supervised fine-tuning stage. Early models demonstrated “impressive reasoning capabilities” but suffered from poor readability and language mixing issues, they wrote.
DeepSeek-R1 was based on and incorporated a small amount of cold-start data and multistage training. The approach improved reasoning performance and readability, achieving results comparable to OpenAI-o1-12171, a generative pretrained transformer that was released last September.
The authors described the reinforcement learning process, emphasizing the role of reward modeling and the challenges encountered, such as reward hacking. Smaller models distilled from DeepSeek-R1 showed notable performance improvements with distilled models using less than 70 billion parameters, compared with the trillions that are used to train the largest AI models.
DeepSeek-R1 achieved high scores on multiple benchmarks and performs well on coding, general knowledge and open-ended generation tasks.
Gartner’s Dekate noted some critical details that he said alarmists have missed. One is that DeepSeek-R1 was trained on a “mixture of experts” model, which comprises multiple neural networks that are each optimized for a different set of tasks. When the model receives a prompt, it routes the task to the neural network best-equipped to process it.
MoE is already widely used, having first appeared in Mistral AI SAS’s Mixtral 8x22B model released last spring. It’s an effective way to scale large AI models efficiently but adds complexity in training, deployment and debugging.
He also disputed claims that DeepSeek is fully open. Though the source code is available on Hugging Face, the training data isn’t. “It is an open weights model, but they also do not disclose the data aspects of their infrastructure,” he said. Open weights refers to an AI model whose trained parameters, or weights, are publicly available.
These reservations shouldn’t detract from the technical accomplishments DeepSeek has documented, Dekate said. They will be quickly adopted by competitors and move the industry forward. “You’re going to see the broader market incorporate some of the resource utilization optimization in these models and use this as a foundation to scale even more,” he said. “Competition is a good thing.”
THANK YOU