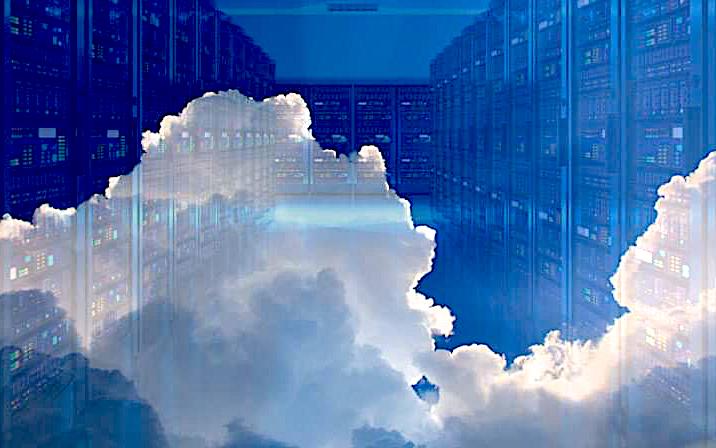
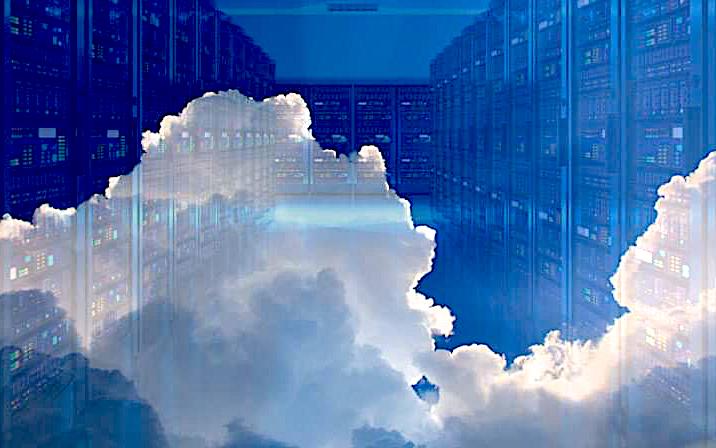
Multiclouds have already arrived in enterprises, thanks to an emerging new kind of networking driven by artificial intelligence.
Intent-based networking is a DevOps paradigm for simplifying, speeding and improving management of multiclouds. This approach involves continuous optimization of availability, reliability, performance, security and other infrastructure policy objectives.
Intent-based networking is not exactly a new management technology, but rather a unified DevOps framework for automating scalable, end-to-end deployment, optimization and control of distributed systems and the applications and workloads running on them. At its heart, this approach refers to tools and techniques for automating software-defined networking across hybrid, edge and other multicloud environments.
Enterprises may implement intent-based networking in various ways, but it generally builds on these core distributed-systems management principles:
To ensure continuous integration and continuous deployment of applications and infrastructure across increasingly heterogeneous multiclouds, enterprises will need DevOps tools that incorporate intent-based networking, which has artificial intelligence at its heart. The tools should leverage AI to enable flexible movement, monitoring, scaling and transparency and the management of infrastructure and application components, data, workflows, metadata and business logic.
Essentially, intent-based networking is an advanced form of AIOps. This refers to an emerging best practice that uses embedded machine learning and other AI models to make software-defined networks continuously self-healing, self-managing, self-securing, self-repairing and self-optimizing. AI’s growing role in multicloud management stems from its ability to automate and accelerate many tasks more scalably, predictably, rapidly and efficiently than manual methods alone.
AIops becomes more completely automated when it uses data-driven statistical algorithms to capture intent as well as automate the downstream orchestration, monitoring and assurance workloads. More multicloud management vendors are integrating AIOps with intent-based networking to enable this level of closed-loop automation that can radically boost the productivity of customers’ information technology staffs.
One might divide the AIOps market into those providers that leverage AI to automate management of multicloud infrastructure versus those that primarily use it to automate development and release of the microservices that run in that infrastructure. One might also organize providers by the extent to which they also automate the upfront intent discovery process in that AI-driven pipeline.
In the infrastructure-focused camp, one must certainly place Cisco Systems Inc. It has implemented intent-based networking across its portfolio of hardware, software and services for multicloud management, distributed data centers and software-defined wide-area networks. It now supports the following key capabilities:
Much of this AIOps functionality is also found in Cisco Crosswork Situation Manager, which embeds AI for intelligent, adaptive, and automated management of hybrid, mesh and other complex cloud deployments.
To a lesser degree, VMware Inc. is also implementing a limited type of AIOps for multiclouds, though it’s not clear whether it intends to provide the automated intent discovery features needed to build out a closed loop. Last August, VMware announced the preview of “Project Magna,” an R&D initiative that’s developing an AI-driven edge-to-edge virtualization environment to power adaptive security in what it calls a “self-driving data center.”
Project Magna relies on AI to be embedded in hundreds of “internet of things” control points and integrates with the adaptive microsegmentation of VMware’s NSX multicloud networking environment. It will leverage edge-node AI to learn normal application behavior, use that knowledge to lock down compute and network resources, and adapt to continuous change in cloud-native application so it can adjust edge-node microservices’ attack surfaces in an automated, nondisruptive fashion.
As for providers that primarily use AI to automate development and release of the microservices for multiclouds, IBM Corp. springs to mind most readily. As can be seen from its recent product announcements at Think 2019, IBM is becoming a multicloud AI DevOps pipeline provider par excellence. At the event, the company launched new solutions for planning, migrating, integrating, securing and managing applications and workloads across any public or private cloud, any on-premises IT environment and any Kubernetes cluster.
In terms of an infrastructure management capability, the company also launched the new IBM Services for Multicloud Management for self-service acquisition and management of IT resources across multiple cloud providers, on-premises environments, private clouds, legacy infrastructure and container environments. This solution includes:
Though IBM Services for Multicloud Management is a strong tool for its core use cases, it stops short of being an AI-driven, intent-based networking offerings. It has a centralized policy definition tool and integrates with Watson AI Engine to interpret and analyze structured and unstructured IT data for multicloud management, but it lacks the ability to automatically infer business intent in order to create policies for multicloud deployment, monitoring and control. Nevertheless, it can support automatic discovery and remediation of multicloud security vulnerabilities, detect suspicious traffic and drive continuous event monitoring, application-aware firewalling, intrusion prevention, anti-malware and URL filtering.
In a broader sense, any infrastructure as code or IaC solution may be used as the foundation for AIops in general or intent-based networking specifically. IaC drives DevOps around the deployment of functional platform components within complex distributed cloud environments. The methodology does so in the same way one manages such application components as code builds, machine images, containers, serverless functions and security code.
As a multicloud management approach, IaC eliminates the need for IT professionals to touch physical IT platforms, access cloud providers’ management consoles, log into infrastructure components, make manual configuration changes or use one-off scripts to make adjustments.
As an alternative to traditional IT change-and-configuration management, IaC involves writing templates — aka “code” — that declaratively describe the desired state of a new infrastructure component, such as a server instance, virtual machine, container, orchestrated cluster or serverless functional app.
Within IT management tooling that leverages underlying DevOps source control, the IaC template drives the creation of graphs of what the cloud infrastructure codebase should look like. The tooling then looks for deficiencies in deployed code and fixes them by deploying the end-to-end code so the end-to-end deployed infrastructure converges on the correct state. Conceivably, the upfront code/policy capture in IaC could be automated with AI.
However, this automated-code-generation capability isn’t yet found in such cloud IaC tools as AWS CloudFormation, Azure Resource Manager, Google Cloud Deployment Manager) or in third-party DevOps vendors whose solutions address diverse public, private, hybrid and multicloud deployments (e.g., Terraform, Saltstack, Juju, Docker, Vagrant, Pallet, CFEngine, NixOS).
IaC is a fast-evolving segment of cloud-native DevOps. For a discussion of IaC’s role in building hybrid serverless applications, please check out this Wikibon note I published late last year.
THANK YOU