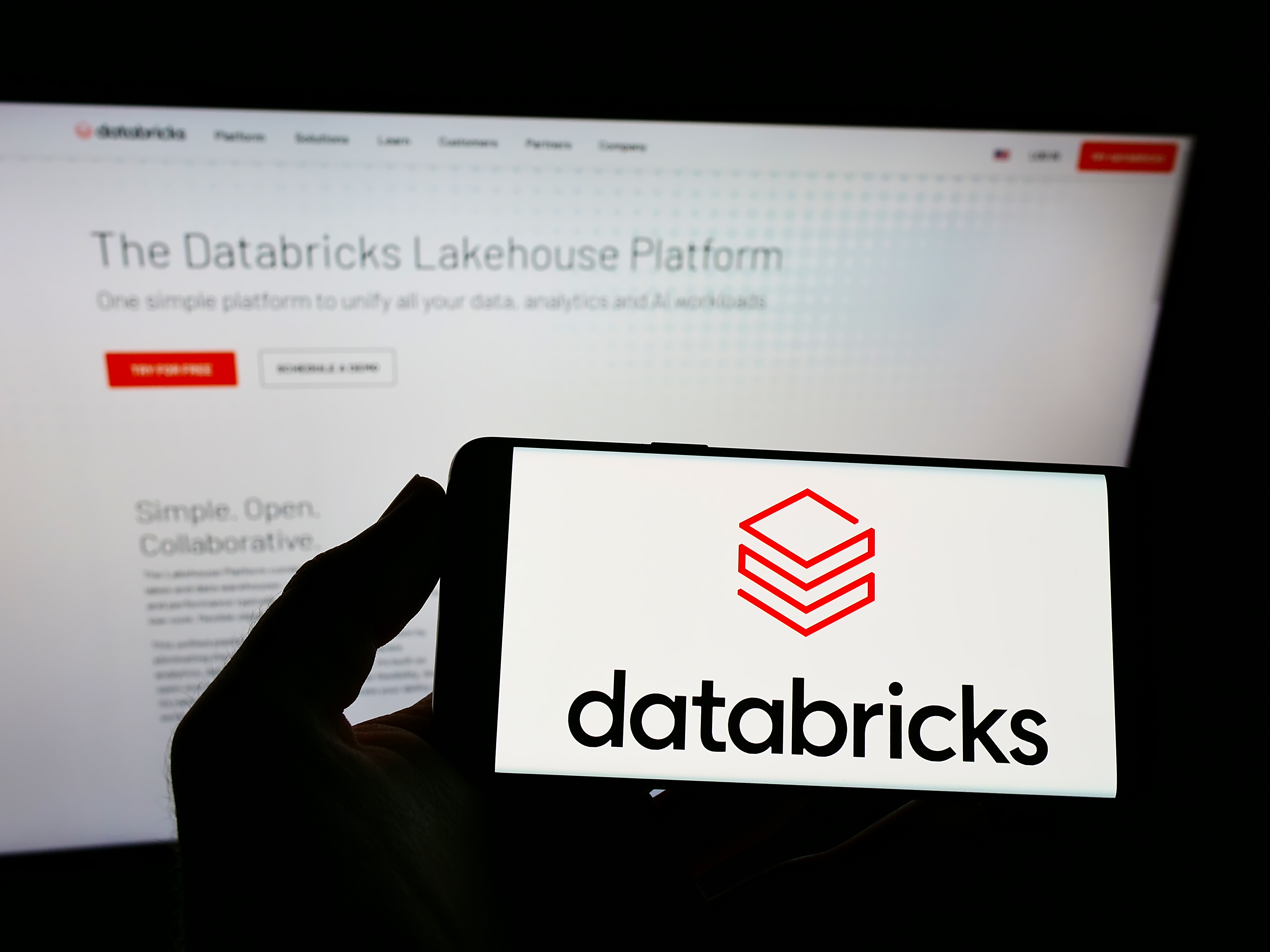
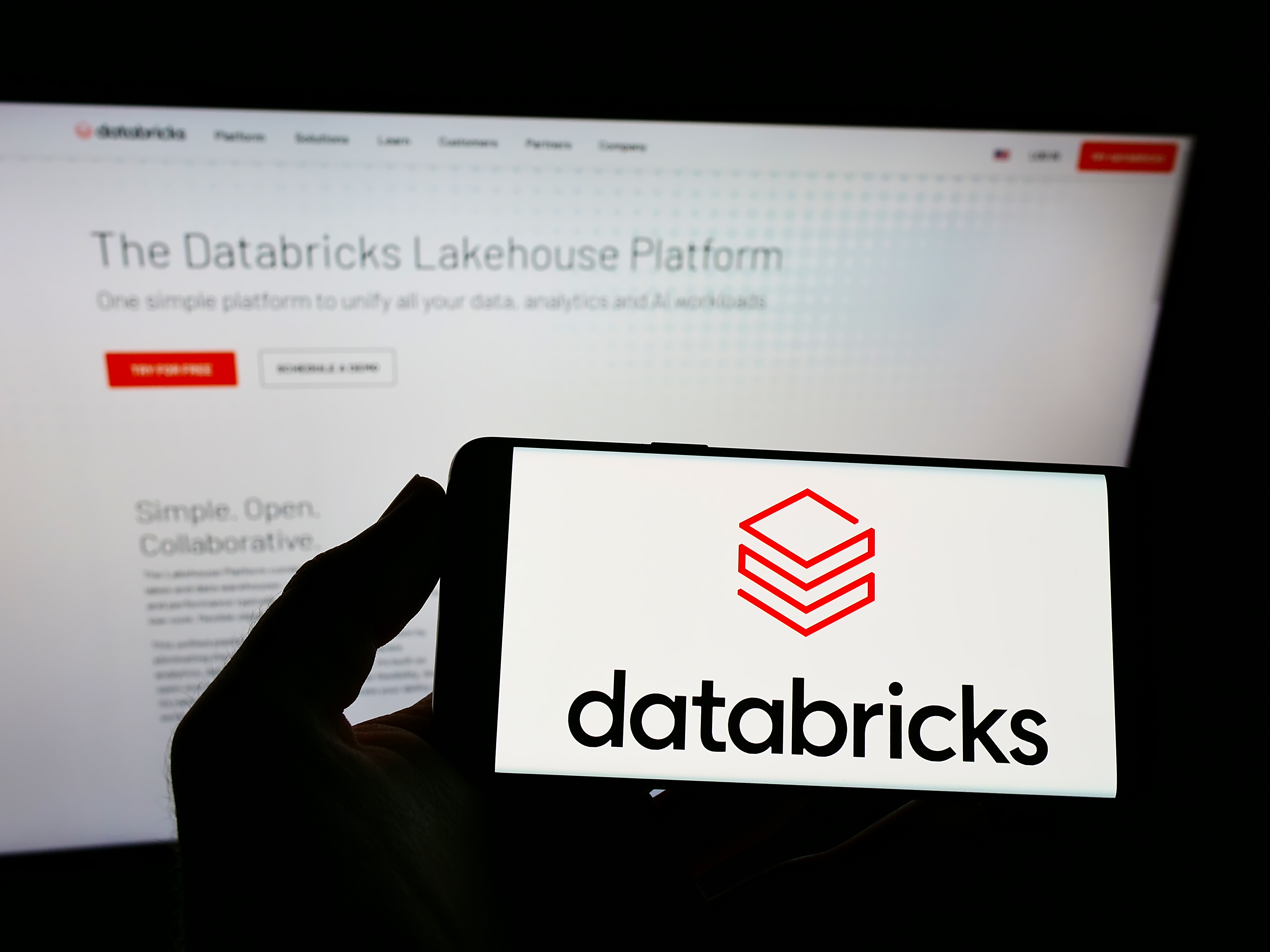
With George Gilbert, Rob Strechay and Andy Thurai
The recent Databricks Data+AI Summit attracted a large audience and, like Snowflake Summit, featured a strong focus on large language models, unification and bringing AI to the data.
While customers demand a unified platform to access all their data, Databricks Inc. and Snowflake Inc. are attacking the problem from different perspectives. In our view, the market size justifies the current enthusiasm seen around both platforms, but it’s unlikely that either company has a knockout blow for the other. This is not a head-on collision.
Rather, Snowflake is likely years ahead in terms of operationalizing data. Developers can build applications on one platform, like Oracle Corp. when it won the database market, that perform analysis and take action. Databricks likely has a similar lead in terms of unifying all types of analytic data – business intelligence, predictive analytics and generative artificial intelligence. Developers can build analytic applications across heterogeneous data, like Palantir Technologies Inc. does today. But they have to access external operational applications to take action.
In this Breaking Analysis, we follow up last week’s research by connecting the dots on the emerging tech stack we see forming from Databricks, with an emphasis on how the company is approaching generative AI, unification and governance — and what it means for customers. To do so, we tap the knowledge of three experts who attended the event, CUBE analysts Rob Strechay and George Gilbert and AI market maven Andy Thurai of Constellation Research Inc..
The three big themes of the event are seen above with several announcements and innovation areas of focus shown beneath each one.
The keynote from Chief Executive Ali Ghodsi was very strong. Co-founder and Chief Technologist Matei Zaharia participated extensively, as did Naveen Rao from MosaicML, and the other co-founders got some good air time. We also heard from JP Morgan Chase & Co. onstage as well as JetBlue and Rivian, and that was just day one, which was followed up by Andreessen Horowitz’s Marc Andreessen and former Google Chairman and CEO Eric Schmidt on day two.
The consensus was that Databricks put on a first-rate program with lots of technical and business meat on the bone. The following points further summarize our views:
That said, we would like to see more integration with other data source types from hyperscale cloud providers. Overall, however, the announcements and the event were compelling.
In our view, the keynote was impressive, presenting a robust vision for future growth and development in key areas. The introduction of LakehouseIQ, along with the acquisition of MosaicML and the continuing maturation of Unity Catalog, puts Databricks in a strong position to maintain its position and compete for incremental share. However, we believe further integration with open-source modeling and other data source types such as cloud providers would make its strategy more inclusive and appealing. Despite some identified areas of improvement, we believe the keynote was overall successful and compelling.
The slide above depicts what we see as Databricks’ emerging tech stack, building on top of previous work we’ve shared in Breaking Analysis. In our view, Databricks has successfully pivoted from what was perceived as a weakness – data management – to a strength, through its harmonization and unification of all data assets and analytic data products.
The following additional points are noteworthy:
In our opinion, Databricks has successfully turned a perceived weakness into a strength by focusing on the harmonization and unification of data assets. With the introduction of LakehouseIQ and Unity, and their move toward gen AI applications, we believe Databricks is shaping a future where the ability to model business entities in familiar language and transparently translate them into technical data artifacts is central. These innovations hold the potential to profoundly transform how businesses interact with and manage their data.
It has been well-documented that the MosaicML acquisition was a mostly stock deal done at Databricks’ previous approximately $38 billion valuation, now believed to be in the low $20 billion range. As such, the actual cost of the acquisition to Databricks was a little more than half of the reported purchase price of $1.3 billion. As with Snowflake’s approximately $150 million acquisition of Neeva Inc., a key motivation of these moves is to acquire talent and tech, which is in short supply.
The acquisition is a strategic move for Databricks. The idea is to offer enterprises the tools to build their own LLMs easily and cost-effectively, using their own data, while making the whole process part of the broader Databricks toolchain and workflow. This strategy could potentially reduce the costs associated with training and running these models. Specifically, while general-purpose LLMs will continue to exist, we believe there is a market for specialized LLMs that are more cost-effective and fine-tuned for specific tasks. Both Snowflake and Databricks are working towards providing this capability focusing on enterprise-class governance and IP protection.
Two other key points:
There is some fear, uncertainty and doubt around loss of control of customer IP, which most nonhyperscale cloud providers want to foster. Hyperscale providers typically provide options to create private instances of LLMs fine-tuned on a customer’s data.
Although the analogies may make each respective company bristle, we see Snowflake as Oracle-like in that it is focused on unifying all forms of data management with the objective of operationalizing analytics. That means not only performing the analysis and presenting the results but actually taking action on the data. This is all with a focus on an integrated experience with a promise of strong and consistent governance.
Databricks we view as Palantir-like in that we see it using LLMs to build a semantic layer on top of Unity’s catalog of all analytic data assets. Most semantic layers have the business rules and logic spelled out programmatically. LakehouseIQ uses an LLM to infer these business-meaningful connections. From the data artifacts, notebooks, dashboards and models it can begin to infer business concepts such as organizational structure or product line hierarchies or a company’s use of a revenue calendar.
Furthermore, the following points are relevant:
Whether perceived or real, many customers have cited that building the data engineering pipelines outside Snowflake (or in Databricks) is more cost-effective. This perception may originate because Snowflake bundles AWS costs in its consumption fees, whereas Databricks does not. As such, customers receiving the Databricks bill may see it as cheaper. It’s also possible that the Snowflake engine is optimized for interactive queries and so carries more overhead for batch pipelines. More research is required to determine the actual total cost of ownership of each environment and that will take time.
Databricks strategically emphasizes the use of Amazon S3 or object-based storage in its architecture, which it positions as advantageous in terms of cost competitiveness. This decision aids them in their relationship with cloud providers as they can sell more capacity at a lower price. In contrast, while Snowflake stages data from S3/object stores, it also leverages block storage along with compute services as part of its cloud services layer architecture. Block storage has proven to be extremely reliable and performant but it is also more expensive.
While perhaps appropriate for many workloads, this makes Snowflake’s underlying architecture comparatively appear more expensive and in times of budget constraints could present headwinds for the company’s consumption model. Snowflake began aggressively addressing cost competitiveness last year by integrating Iceberg tables and further pushing into S3. This cost reduction strategy was a major focus during the initial keynotes, signaling Snowflake’s commitment to making its platform more affordable.
As we reported last week, we suspect Snowflake is strategically banking on Nvidia Corp.’s stack by wrapping it into its container services. We posited that it was Snowflake’s intention to leapfrog existing ML/AI tool chains, a field where Databricks historically excels, and advance directly to the unsupervised learning for generative AI models. However, Databricks appears to be taking the same leapfrogging approach…disrupting its own base before the competition does so.
Snowflake in our view definitely sees this as an opportunity for a reset, as we inferred from Senior Vice President of Product Christian Kleinman’s interview with theCUBE and Nvidia. Meanwhile, Databricks has made a significant pivot in the last six months, particularly with the MosaicML acquisition. As Ali Ghodsi stated, MosaicML “just works” – you simply fill out a configuration file and the system trains the model.
Though not everyone will want to train their own models, it’s notable that the number of customers using Hugging Face transformers went from 700 to more than 1,500 on the Databricks platform in the first six months of the year. Moreover, the company shared that its consumption of GPUs is growing by 25% month over month. This indicates a substantial demand for building and running specialized models. Databricks aims to cater to this demand by being the most accessible and user-friendly platform for training and fine-tuning. This shift in strategy by Databricks is notable and has merit in our view.
Both Databricks and Snowflake are driving toward a unified platform but significant differences remain, in a large part related to the audiences they originally served – that is, data scientists versus BI professionals. We often discuss these two firms as on a direct collision course, but the reality is their paths to unification are different and we believe the market is large enough such that both firms can thrive.
To be specific, Databricks was the center of gravity for building data engineering pipelines out of semistructured clickstream data from mobile apps and websites. Data scientists and ML engineers used this refined data for building ML models that might predict customer behavior. Spark’s support for procedural languages such as Python, libraries for working with semistructured data, and integrated ML tools made it the natural home for this type of development.
Snowflake’s SQL-only roots and peerless support for interactive SQL queries made it the natural home of data engineers, data analysts and business analysts building business intelligence applications. But Snowflake’s more powerful DBMS engine has let it add support for multimodel transactional workloads as well as procedural languages such as Python. It still has much work to do in capturing the hearts and minds of the Python community doing AI/ML.
A big part of Databricks’ marketing narrative is to position Snowflake as an outdated data warehouse. Although Snowflake dramatically simplified enterprise data warehouses and unleashed the power of the cloud by separating compute from storage, its data cloud vision and application development framework are creating new markets well beyond traditional enterprise data warehouse markets.
Nonetheless, Databricks spent considerable time at its event discussing how it is reimagining its data warehouse engine, Databricks SQL. The company had Reynold Xin, its co-founder and chief architect, onstage talking about how it’s reconceiving the data warehouse by eliminating tradeoffs between query optimization (such as speed), costs and simplicity.
Its approach is to circumvent decades of research on query optimization by collecting years of telemetry on the operation of Databricks SQL. It uses that data to train AI/ML models that make better optimization decisions than the assumptions embedded conventional engines. His argument was that Databricks has figured out how to give you all three with no tradeoffs. He mentioned some unnamed company’s Search Optimization Service (he was, of course, talking about Snowflake) and how they were expensive and forced to make such tradeoffs.
One other area Databricks stresses as a competitive advantage is its openness. Here’s what Databricks’ Zaharia on theCUBE with John Furrier addressing this topic.
One of the big things we’ve always bet on is open interfaces. So that means open storage format so you can use any computing engine and platform with it, openAPIs like Apache Spark and MLflow, and so on because we think that will give customers a lot more choice and ultimately lead to a better architecture for their company. That’s going to last for decades as they build out these applications. So we we’re doing everything in that way where if some new thing comes on, that’s better at ML training than we are or better at SQL analytics or whatever. You can actually connect it to your data. You don’t have to replatform your whole enterprise, maybe lose out some capabilities you like from Databricks in order to get this other thing, and you don’t have to copy data back and forth and generate zillions of dollars of data movement.
Watch Matei Zaharia discuss Databricks’ philosophy on open interfaces.
Databricks believes it has an edge over Snowflake relative to its open-source posture. It has based its technologies, such as Delta Lake and Delta Tables, on open-source platforms such as Apache Spark and MLflow. During the event, Databricks revealed two additional contributions to the open-source community. The intention seems to be to create a barrier against Snowflake by championing the benefits of open-source technology over Snowflake’s closed-source system.
Databricks isn’t just promoting open source but also provides the value-add of running the software for users as a managed service. Its proposition is to let users store their data in any format (Delta tables, Iceberg, Hudi) and run any compute engine on it, which it positions as currently a more open approach than what Snowflake offers.
The number of downloads for Databricks’ open-source offerings is substantial. Spark has a billion downloads per year, Delta Lake has half a billion, and MLflow has 120 million downloads per year.
However, Snowflake would argue that it provides access to open formats, it commits to open-source projects, and it supports a variety of query options. Ultimately customer spending will be the arbiter of how important the open posture is to the market.
To add some context here, Enterprise Technology Research began tracking Databricks’ entry into the database/warehouse space only recently. When new products are introduced it often takes several quarters or more to collect enough critical mass in the survey base and that has been the case with Databricks in the database market. The chart below shows Databricks’ customer spending profile for the Database/Data Warehouse sector in the ETR data set.
The graphic shows the granularity of Net Score, ETR’s proprietary methodology that tracks the net percentage of customers spending more on a platform. The lime green indicates new logos, the forest green represents the percent of existing customers spending 6% or more relative to last period, the gray is flat spend, the pink is spending down 6% or worse, and the red is the percentage of customers churning.
Subtract the reds from the greens and you get Net Score, which is shown on the blue line. Databricks in this space has a very robust Net Score in the mid-60s. Note that anything above 40% we consider highly elevated. Moreover, while the company’s entrance into this space is relatively recent, the survey sample is roughly N=170+.
The yellow line is an indicator of presence in the data set, calculated by the N divided by the total N of the sector. So as you can see, it’s early days for Databricks in this market — but its momentum is strong as it enters the traditional domain of Snowflake.
The ETR data set uses a taxonomy in order to enable time series tracking and like-to-like comparisons. The intent is where possible to make an apples-to-apples comparison across platforms and that requires mapping various products into taxonomical buckets. We stress this point because vendor marketing rarely allows for simple mappings. As an example, ETR doesn’t have a “Data Cloud” category, but it has begun to track Snowpark and Streamlit, which allows us to gauge the relative strength of various platforms and force our own comparisons across taxonomical buckets.
With that in mind, the following chart shows the same Net Score granularity as the previous chart for Snowflake.
Several points are notable:
The other main takeaway is Snowflake’s presence in this market is maturing with a much longer history than Databricks. Its N is 50% larger than that of Databricks as seen in the yellow line above.
The reverse case is similar. In other words if you model Snowflake’s presence in Databricks’ wheelhouse the delta is even more pronounced. In other words, Snowflake appears to have more ground to make up in the world of data science than Databricks seems to have in the data warehouse sector. That said, data management is perhaps a more challenging nut to crack.
Databricks and Snowflake have contrasting strategies in their approaches to data management. Initially, Databricks functioned like Informatica, developing data engineering pipelines that converted raw data into normalized tables that data scientists used for ML features. Many companies used both Databricks and Snowflake for analytics and interactive dashboards.
However, Databricks has now evolved its platform to encompass both data engineering and application platform functions, similar to what WebLogic was known for in the era of web applications in the on-premises world. Although Databricks is not yet a database management system that handles operational and analytic data, it’s leveraging its data engineers and data scientists to create analytic apps. The combination of Unity and LakehouseIQ within its platform aims to make Databricks an application platform, akin to what Palantir has achieved.
In contrast, Snowflake has emerged as a dominant database management system akin to Oracle. It unifies all data types under a single query and transaction manager. Hence, while Databricks is expanding its range to become an analytic application platform, Snowflake continues to strengthen its position as a powerful DBMS. It still has work to do in building the application services that make development of operational applications much easier.
Data application platforms like Databricks and Snowflake are integral in our increasingly data-driven world. However, there seems to be a shift in the perception of these platforms:
Both Snowflake and Databricks have much work ahead to close gaps:
When it comes to model creation, model maintenance, model management and data governance, Databricks’ focus is notable. An upcoming challenge for these platforms will be managing privacy and regulations when deploying models, especially across different jurisdictions. This area, currently not completely addressed by either platform, will become increasingly important as more large language models move into the production phase.
On balance, the Databricks event impressed us and noticeably elevated our view of the company’s position in the market. The fast-paced nature of the industry means things can change rapidly, especially when large established players such as AWS, Microsoft, Google and Oracle continue to invest in research and development and expand their respective domains.
Many thanks to Alex Myerson and Ken Shifman on production, podcasts and media workflows for Breaking Analysis. Special thanks to Kristen Martin and Cheryl Knight, who help us keep our community informed and get the word out, and to Rob Hof, our editor in chief at SiliconANGLE.
Remember we publish each week on Wikibon and SiliconANGLE. These episodes are all available as podcasts wherever you listen.
Email david.vellante@siliconangle.com, DM @dvellante on Twitter and comment on our LinkedIn posts.
Also, check out this ETR Tutorial we created, which explains the spending methodology in more detail. Note: ETR is a separate company from Wikibon and SiliconANGLE. If you would like to cite or republish any of the company’s data, or inquire about its services, please contact ETR at legal@etr.ai.
Watch the full video analysis:
All statements made regarding companies or securities are strictly beliefs, points of view and opinions held by SiliconANGLE Media, Enterprise Technology Research, other guests on theCUBE and guest writers. Such statements are not recommendations by these individuals to buy, sell or hold any security. The content presented does not constitute investment advice and should not be used as the basis for any investment decision. You and only you are responsible for your investment decisions.
Disclosure: Many of the companies cited in Breaking Analysis are sponsors of theCUBE and/or clients of Wikibon. None of these firms or other companies have any editorial control over or advanced viewing of what’s published in Breaking Analysis.
THANK YOU